This week (July 28 - August 1, 2019) I attended the 15th US National Congress on Computational Mechanics (USNCCM) in Austin, TX. I was pleased to be invited to present a talk entitled "Toward a Self-Aware Aircraft: Data-Driven Decisions, Adaptive Reduced Models, and Digital Twins" in the minisymposium on "Model Order Reduction for Computational Continuum Mechanics". Details of my presentation, as well as a link to the presentation slides, is given below. Thank you to the conference organizers, and to the minisymposium chairs for the opportunity to present, and for hosting a great conference.
Slides: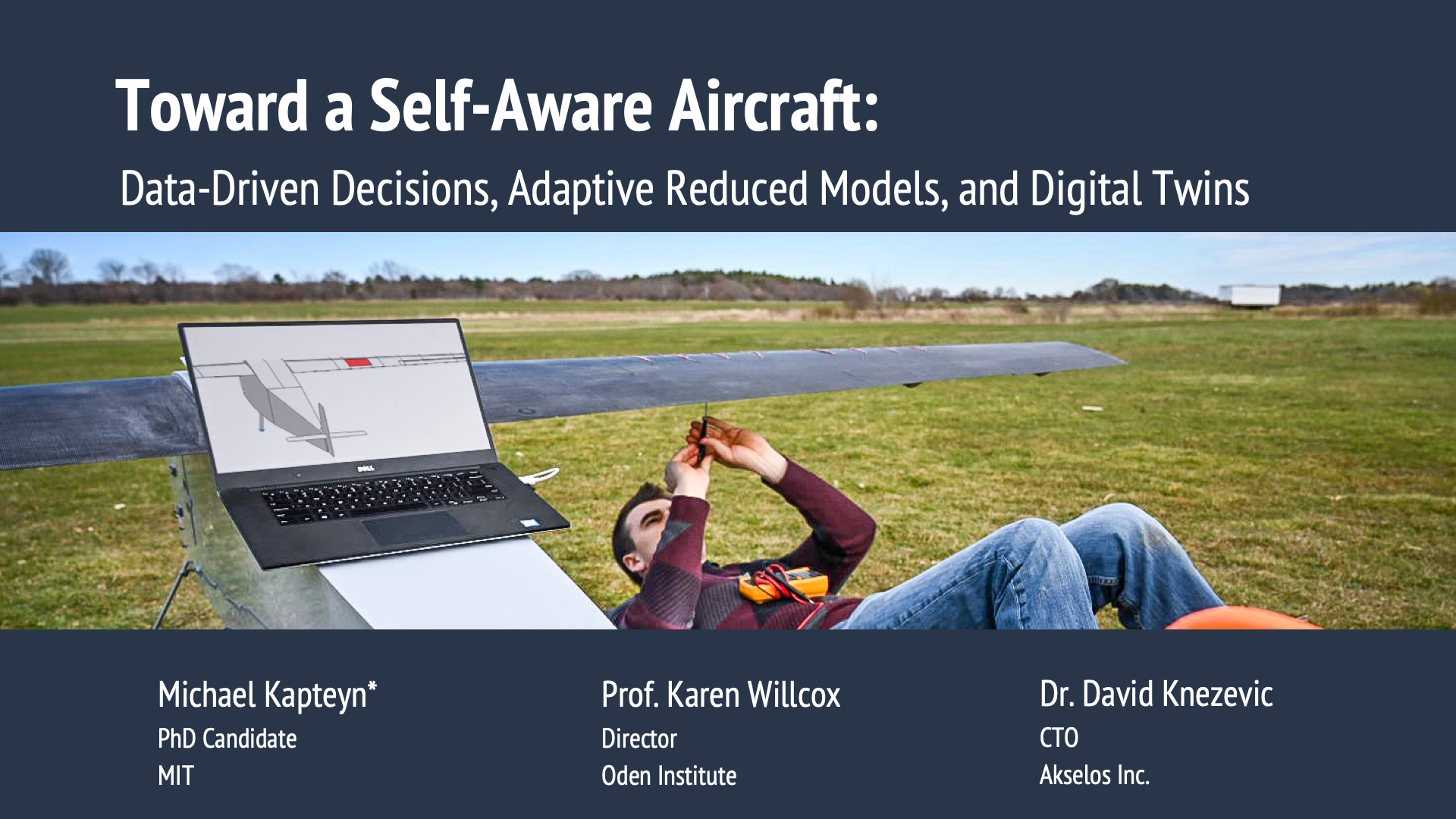
Abstract:
This talk presents a computational framework that combines reduced-order models (ROMs) with online sensor information in order to enable the next generation of self-aware unmanned aerial vehicles (UAVs). A self-aware UAV is one that maintains knowledge of its own internal state and acts accordingly[1]. The internal state we consider is the vehicle’s structural health. The vehicle uses sensor data to detect changes in its structural health, and responds intelligently by adapting the way it performs. This allows the vehicle to fly aggressively when it is healthy, while avoiding structural failures by becoming more conservative as it degrades. Such capability has the potential to improve performance over the full vehicle lifecycle, while also reducing structural health monitoring and maintenance costs.
We create a Digital Twin of the UAV, using a combination of physics-based and empirical models that represent the key aspects of the vehicle’s performance. A core part of this Digital Twin is a component-based ROM of the aircraft structure[2]. This structural model is evolved and adapted over the lifecycle of the vehicle, such that it reflects the vehicle’s current structural health after each mission. The component-based ROM paradigm provides flexibility in structural defect modeling, while also permitting efficient recalibration in the face of dynamic data. The Digital Twin is used to construct a library of ROMs that represent possible future structural states. Each entry in the library is mapped offline to a corresponding estimate of vehicle capability. This library is used in flight, as online sensor data is assimilated, to rapidly classify the evolving structural state over time. The near real-time estimation of the vehicle’s structural state enables dynamic mission re-planning in response to in-flight events such as damage to the UAV’s wing[3].
This talk will focus on computational aspects of this project, which also includes a 12-foot wingspan flight-test vehicle being constructed by the Willcox Research Group and Aurora Flight Sciences.
[1] Allaire, D., G. Biros, J. Chambers, O. Ghattas, D. Kordonowy, and K. Willcox. “Dynamic Data Driven Methods for Self-Aware Aerospace Vehicles.” Procedia Computer Science 9 (2012): 1206–1210.
[2] Ballani, J., Huynh, D. B. P., Knezevic, D. J., Nguyen, L., & Patera, A. T. (2018). A component-based hybrid reduced basis/finite element method for solid mechanics with local nonlinearities. Computer Methods in Applied Mechanics and Engineering, 329, 498-531.
[3] Singh, V., & Willcox, K. E. (2017). Methodology for Path Planning with Dynamic Data-Driven Flight Capability Estimation. AIAA Journal, 2727-2738.